Generative AI overview
As we journey across the echelons of technological progress, one avenue is crystallizing as a standout protagonist in reshaping our collective future - Generative Artificial Intelligence (AI). This transformative genre of AI is redefining the boundaries of innovation, unlocking unprecedented potential across various sectors, be it cloud computing, cybersecurity, or the broader ethical and regulatory landscape. Embedded deep into the foundation of Generative AI are complex machine learning models like Generative Adversarial Networks (GANs) and Variational Autoencoders (VAEs), essentially serving as its heartbeat. Their understanding paves the way towards unraveling how Generative AI crafts its magic.
Understanding Generative AI
Understanding Generative AI: Essential Concepts and Models
Generative AI, sometimes also known as generative artificial intelligence or generative modeling, is an exciting branch of machine learning. It predominantly focuses on unsupervised and semi-supervised learning methodologies to produce new content similar to previously available data. The generated output can range from natural language texts, images, music, or any other form of digital content.
A critical basis of Generative AI is probability distribution. Predictive AI models, in general, are trained to recognize patterns from the input data, and subsequently, make future predictions based on that data. On the other hand, Generative AI models instead focus on understanding the underlying data distribution and thus, generate artificial instances from that distribution.
A few popular methods utilized in Generative AI today are:
- Generative Adversarial Networks (GANs): GANs are neural networks that learn to generate new data similarly to the original dataset. GANs have two primary components – a Generator and a Discriminator. The Generator is trained to generate data meant to mimic the original data while the Discriminator validates the authenticity of the generated data by distinguishing between the original and artificial data. This adversarial interaction leads to the generator improving its capacity to create more genuine data, whereas the discriminator improves its capacity to differentiate the generated data from the original data.
- Variational Autoencoders (VAEs): VAEs follow an encoding-decoding approach to generative modeling. A VAE utilizes an encoder to convert a data instance into a compressed latent-space representation. Subsequently, this condensed representation is then regenerated back into the original data instance by a decoder. This two-step approach allows a VAE to generate new data closely resembling the original data while maintaining significant diversity.
- Autoregressive Models: Autoregressive models are a type of statistical models used for predicting future data based on previous data points. In this context, autoregressive models like PixelRNN and WaveNet generate a single element at a time and recursively use generated elements to create new ones.
These primary generative models have a wide range of applications in the real world. From generating synthetic medical images to training anomaly detection systems in cybersecurity, they are gaining enormous interest in the AI research community. They play a pivotal role in enhancing virtual realities, creating digital art, improving video game characters, and much more.
However, as powerful and as exciting as these models happen to be, they also represent critical challenges. The balance between diversity and quality in generated content, computational resource constraints, and ethical issues around misuse are some of the areas that need careful deliberation.
Stepping into the era of unsupervised and semi-supervised machine learning, understanding and exploring Generative AI can unlock a new dimension of possibilities in the technological world of AI. With continuous research and development, the applications of these concepts and models can only stand to expand substantially in the future.
Generative AI in Cloud Computing
Entering into the dynamic field of Generative AI, its contribution to cloud computing emerges as a critical point of discussion. Harnessing the power of these intelligent algorithms, the technology augments the cloud computing environment by expanding system capabilities, introducing novel problem-solving strategies and even, reshaping the chain of product development.
A key facet of this integration is the operational augmentation that Generative AI brings to cloud operations. Utilizing GANs, VAEs, and Autoregressive Models, the system's operational efficiency is escalated through automation. For instance, these AI models can be embedded within DevOps pipelines to enhance software testing processes. With Generative AI algorithms creating plausible, diversified test data in real-time, systems can be probed more intensely and in varied manners, amplifying the comprehensiveness of the testing process.
Generative AI, being renowned for its advanced data synthesis capabilities, has bolstered cloud security measures. It has amplified Intrusion Detection Systems (IDS) in cloud infrastructures. By generating legitimate network traffic scenarios, it strengthens the IDS's capabilities in identifying malicious activities, consequently enhancing the erudition of the algorithm concerning intricate hacking techniques.
Furthermore, these models are now contributing significantly to refining cloud resource optimization. Traditional methods often fail to anticipate and respond accurately to fluctuating demands posed by traffic. Generative AI, with its probabilistic framework, can accurately emulate user behavior. This empowers cloud providers to construct superior strategies for resource allocation, reducing operational costs while improving service performance and reliability.
Interestingly, one of the fastest-growing uses of Generative AI within cloud computing is in the development of synthetic datasets. As privacy regulations tighten across jurisdictions, utilizing actual customer data for training and development purposes is fraught with risk. Generative AI algorithms solve this problem by creating synthetic datasets that resemble actual data without the risk of exposing real customer details, thereby transforming data privacy practices within cloud infrastructures.
As our reliance on cloud services outpaces the growth of conventional data centers, edge computing has emerged as a significant trend. Generative AI shapes this trend through intelligent modeling of resource allocation at the edge, ensuring optimum use of limited resources. Moreover, leveraging Generative AI capabilities in predictive modeling can efficiently manage network load, thereby improving service latency and bandwidth usage, which are crucial factors in edge computing.
Despite the opportunities presented by integrating Generative AI within cloud computing, it doesn't come without challenges. Controlling the randomness of generated outputs, preserving privacy in the generated samples, and ensuring responsible use of AI are all potential hurdles. Moreover, the battle against malicious uses of such technologies, such as deepfakes, takes center stage in the discussion around Generative AI.
Bearing these considerations in mind, it's undeniable that Generative AI is changing the face of cloud computing. It's providing the tools to learn from complex data, innovate faster, and deliver superior services. As mainstay in our evolving digital age, expect Generative AI to increasingly define the path that cloud computing will take in the coming years.
Generative AI and Cybersecurity
The Impact of Generative AI on Cybersecurity
Having explored the way Generative Artificial Intelligence (AI) functions and its numerous applications, it is crucial to delve into its impact on the constantly evolving domain of cybersecurity. As cybersecurity threats continue to increase in complexity, the integration of Generative AI has become of paramount importance to counteract these intricate virtual perils.
The use of Generative AI in cybersecurity fundamentally serves two key objectives. Firstly, it enhances the security of systems by smartly identifying potential vulnerabilities and recommending actions. Secondly, through the generation of artificial but realistic data, it simplifies the process of training and optimization of cybersecurity models.
Generative AI finds a particularly fascinating application in Intrusion Detection Systems (IDS). An IDS monitors network traffic, detecting suspicious activity and alerts the system or network administrator. By leveraging Generative AI techniques such as GANs, cybersecurity professionals can significantly improve the precision of anomaly detection tools. They can generate realistic network traffic to test and enhance the system's detection abilities, resulting in a more secure and reliable digital ecosystem.
Additionally, through the exploitation of GAN’s powerful capabilities, cybersecurity firms can generate synthetic cyber-attack patterns. These patterns, which mimic real attacks, can be executed in a controlled environment, allowing cybersecurity professionals to understand how to proactively harden systems and networks against genuine assaults.
Equally exciting is the potential of Generative AI to enhance privacy protection measures. Privacy is a significant concern when dealing with massive data flows in cloud infrastructures, especially in multi-tenant environments. Generative AI can create synthetic data sets that retain the original data's statistical characteristics but do not compromise personal information, catering to the need for privacy-preserving data sharing.
The deployment of Generative AI is not limited to security and privacy protection. It also plays a critical role in resource optimization in cloud computing services. By applying the probability-based approach inherent in Generative AI models, cloud service providers can improve the assignment of resources across different workloads, leading to better performance and reduced costs.
Moreover, Generative AI is making its mark on the increasingly prevalent field of edge computing. By generating lightweight models based on cloud-trained AI, edge devices can locally infer and make decisions, thereby reducing the dependency on constant cloud connectivity and greatly enhancing resilience against network attacks.
However, the integration of Generative AI in cybersecurity is not without its challenges. These include a lack of interpretability of predictive models, the need for robustness against algorithmic attacks, and the necessity of privacy preservation during learning. Nonetheless, with continuous research and development, these obstacles can be overcome, paving the way for a new age of cybersecurity underpinned by Generative AI.
As we continue to advance technologically, the role of Generative AI in defining the future of cloud computing is undeniable. With its crucial contributions to cybersecurity and its far-reaching implications for the digital world, Generative AI will offer a much safer and smarter future for information technology. Despite the challenges, with the right tools and understanding, the marriage between Generative AI and cybersecurity holds the promise of a more secure electronic frontier.
Ethical and Regulatory Aspects of Generative AI
Transitioning towards ethical and regulatory challenges, Generative AI, given its innate power to generate, augment, or refine data, opens vast opportunities but also uncovers a Pandora's box of ethical and regulatory concerns. These encompass issues such as copyright, attribution, privacy, and consent.
For instance, using Generative AI to create new content, such as a book or an artwork, raises the question of copyright ownership- would it rest with the AI developer, the machine or the individual feeding the data?
Furthermore, Generative AI opens a new front in the battle for privacy. If, for example, a synthetic dataset mirrors real-world data too closely, it may inadvertently reveal real-world individuals' private information. The data privacy and data protection regulations at present, such as the EU's General Data Protection Regulation (GDPR), or the California Consumer Privacy Act (CCPA), might need to evolve to better accommodate threats posed by Generative AI.
AI Generated Fake (Deepfake) content, made possible by Generative AI’s technical advancements, have proven difficult to navigate legally and ethically, with their abilities to create astonishingly realistic, yet deceitful, audiovisual content. It highlights the issue of consent as well—has the AI obtained permission from all relevant parties involved in generating new data or modifying existing data?
As an answer to some of the ethical and legal issues, techniques like Differential Privacy are being applied to ensure that Generative AI respects privacy while still producing useful outputs. It adds 'noise' to the data to maintain individual data points' anonymity, helping uphold privacy. In addition, regulatory initiatives such as guidelines for 'Trustworthy AI', as provided by EU's High-Level Expert Group on AI, anchor-wide values such as transparency, fairness, and accountability.
However, the solution lies beyond technology and regulation. Open communication among stakeholders, including technical experts, lawyers, ethicists, and the users themselves, is essential to understand and address these issues more comprehensively.
Regarding Cybersecurity, Generative AI introduces two risks. Firstly, hackers could use these technologies to launch sophisticated attacks. For instance, using GANs to create realistic phishing emails, cybercriminals could trick systems into believing they are genuine. Secondly, the extensive computational resources required by these advanced AI models might be exposed to 'crypto-jacking' attacks, where hackers exploit the resources to mine cryptocurrencies.
In response to these risks, Cybersecurity teams need to stay ahead by incorporating Generative AI into their defense strategies. They can use Generative models to improve intrusion detection systems and simulate cyber-attacks for testing and training purposes.
In summation, while Generative AI exposes several ethical and regulatory challenges, collaborative efforts focusing on building robust legal frameworks, promoting ethical use and creating adaptive defenses, can harness these groundbreaking technologies to our advantage.
Future Prospects of Generative AI
Evolving Landscape of Content Generation with Generative AI
As digital consumption continues to rise, Generative AI will significantly disrupt content-generation channels. Realistic AI-generated text, images, and even audio-visual content will start surging across digital spaces - from personalized ad campaigns to unique music compositions. Similarly, demystifying complex data through generative AI can deliver discernible graphs or images, amplifying data representation and understanding. Notably, decisive strides have been made regarding AI-generated original artworks and literary pieces, prompting reevaluation of copyright ownership and attribution norms.
Preserving Privacy Amidst Progress
Generative AI continues to raise key data privacy questions, especially around consent and usage rights of source material. As it maps patterns within original data to create new content, ensuring protection of sensitive source data or data directly linked to individuals becomes paramount. Especially with generative AI applications in healthcare, such as illness prediction or therapy planning, introducing differential privacy mechanisms will be critical. Here, machine learning algorithms generate synthetic datasets that closely resemble original ones but cannot be traced back to individual records, thereby protecting individuals' privacy.
Trustworthy AI – a Regulatory Imperative?
Inspired by the GDPR’s ‘right to explanation’, guidelines for trustworthy AI, emphasizing transparency, fairness, and non-discrimination are already being conceived. Codes of ethics centered around 'human oversight' and 'technical robustness' could guide generative AI application and usage. Open discussions among academia, businesses, governments, and civil society stakeholders could shape these evolving norms and ensure that generative AI meets societal needs without compromising on values.
The Cybersecurity Implications of Generative AI
As enterprises and governments exploit generative AI's potential, malevolent players are not far behind. Phishing and spear-phishing attacks No multi-day large vocabulary test taking (speech to speech) have already leveraged AI-like capabilities to impersonate trusted entities and trick users into sharing sensitive information or transferring money. Generative AI could exacerbate these threats, making the impersonations nearly indistinguishable from real communications.
Crypto-jacking, another growing digital risk area, could also be magnified. Cybercriminals could train generative AI models on high-performing cryptocurrency mining code, with the generated code then inserted into unsuspecting users' systems.
Mobilizing Cybersecurity Teams Against the Generative AI Threat
The integration of generative AI into cybersecurity practices offers a timely counter-strategy. Generated synthetic cyber-attack patterns can strengthen system defenses, guarding critical digital infrastructure against increasingly sophisticated cyber assaults. Generative AI’s ability to create high-quality, realistic content and stimuli can also be used for rigorous cybersecurity team training, empowering them to counter and mitigate AI-propelled cyber threats.
Building a new paradigm with Generative AI
Emerging technological paradigms like Internet of Things (IoT), Blockchain, and Web3 would also exploit generative AI capabilities. Edge computing, a distributed computing paradigm that brings computation and data storage closer to the location where it is needed, can be significantly enhanced by generative AI algorithms. And generative AI, when integrated with data engineering, DevOps, and other core IT functions, can greatly enhance IT efficiencies across on-premises and cloud infrastructures.
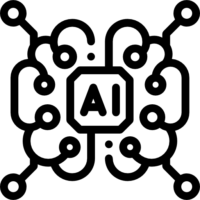
The promise of Generative AI lies within its ability to drastically hone the operational efficiencies, unfold novel avenues for product development, and fundamentally redefine customer experience. However, the terrain of Generative AI is not without its share of precipices. Ethical conundrums, cybersecurity threats, and regulatory challenges present contrastive facets of this burgeoning technology that need an intricate understanding. As the future unfurls, it is only through acknowledging and addressing such clinical issues, can we ensure the responsible harnessing of Generative AI's behemoth potential across industries and sectors. The future belongs to those who not only dare to dream but also arm themselves with the expertise to navigate the complexities of such breakthroughs.